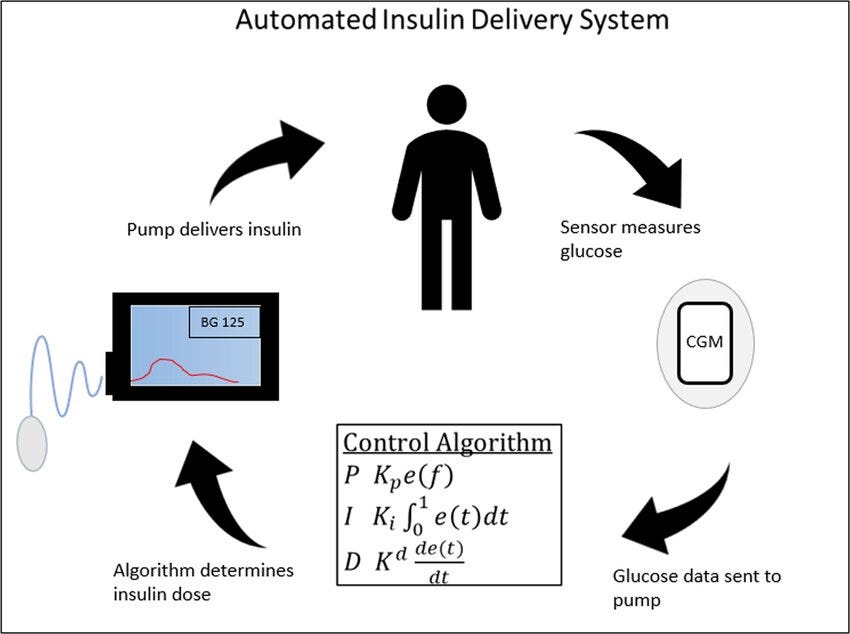
Listen to this really fun AI-generated audio summary of this article.
Automated insulin delivery (AID) systems—also called “closed-loop” systems—operate on the premise that they can assume control of insulin administration by applying mathematical equations to evaluate glucose patterns from a continuous glucose monitor to estimate the amount of insulin needed to maintain glucose levels.
The more than obvious benefits are (1) relieving the human of stress (which should not be discounted), and (2) potentially achieving better glycemic control than individuals can do on their own.
Indeed, these two points have already been demonstrated by a very large number of T1Ds using both existing commercials systems and DIY (do it yourself) kits that are passed between members of affiliate groups. Medical literature is rife with success cases, clinical trials, retrospective studies, and social media accounts of people praising their success.
So, what’s the problem?
Well, in the spirit of this newsletter, it’s not that simple. There are two factors to consider. First, not everyone who try these systems is “successful,” with some subgroups actually doing worse than they did before using AID systems. Second, the definition of “success” is a bit problematic.
All of that is covered in my article, “Benefits and Risks of Insulin Pumps and Closed-Loop Delivery Systems,” which is a prerequisite to reading this article. I don’t recommend trying to digest this article without reading that first, as it teases apart the medical literature to discern exactly who benefits the most, who does worse, and those who see no change at all.
To summarize, the greatest beneficiaries of AID systems are those who cannot or will not manage T1D on their own, such as children (up to mid-20s), the elderly, and those with particular mental health considerations. Those individuals typically have A1c’s well north of 9%, and typically would pay very little attention to their daily regimen. Such people can achieve A1c levels between 7-8%, which is definitely a clear benefit.
Virtually all of the medical literature cited by advocates profile these particular groups, especially children and people up to roughly 26 years old. Their bodies are under rapid change, and they are also young and inexperienced with their T1D, so they haven’t gained the empirical experience with manual glycemic control, nor do most of them have the maturity to do so. Perhaps most importantly, they haven’t developed the intensive self-discipline required for ideal self-management, such as watching CGM data and knowing when and how to dose in anticipation of food, exercise and other events.
Hey, T1D is highly complex and difficult, even for most adults. Kids have their focus on other things in life. (Ah, to be young.)
For all these reasons, young people benefit the most from AID systems, while adults can also benefit if they are also unable or unwilling to apply the self-discipline required glucose control.
But, as the article points out, it’s also not that simple. There are those who love AID systems for the sole reason that it relieves them of that self-discipline. Manual insulin administration and constant CGM monitoring can be a huge mental burden.
And therein lies the grey area between whether AID systems are actually beneficial. It’s where the trade-off lies between convenience and glycemic control. Studies cited in that article show that those who are more engaged with their T1D—who have developed the self-discipline—outperform AID systems and achieve much healthier outcomes.
This is not a small or insignificant group of people. This 2018 study by the T1D Exchange collected data from over 21,000 subjects who used a combination of pumps and MDI (with and without CGMs), shown in the following figure:
The researchers wrote that “there isn’t a substantial difference between A1c outcomes for those who manually take insulin injections compared to those who use pumps” when the users are over the age of 26 and use CGMs. In fact, CGM+MDI users slightly outperformed CGM+pump users.
The last thing to consider is whether AID systems are achieving “healthy” levels of glycemic control. True, achieving an A1c of 7% is obviously better than anything north of that, and many AID users can achieve it. But this is not—and should not—be interpreted as “healthy.” Speaking in absolute terms, health outcomes vastly improve as A1c levels drop below 7%, as measured by all-cause mortality, lipid profiles, cardiac events and strokes, liver and kidney disease, nerve damage, and a large number of other complications. All these are vastly reduced when A1c levels drop progressively lower from 7% to 6.5% to 6%. (See my article, “T1D and Health: How Long Will You Live?”)
Closed-loop advocates say that AID systems are getting better, which may have been true for the past couple years, but the real questions are: What is that maximal level of efficacy? What challenges do they face? What are the alternatives?
That’s what this article covers.
Three key barriers for AID systems
According to peer-reviewed medical literature published over the last 15 years (cited later in this article), AID systems face three key hurdles:
Keeping glucose under control is a highly complex process that has enormous variability, even among healthy non-diabetic individuals. Simulating that organic process is not possible without testing for—and reacting to—far more hormonal activity than merely glucose. As will be illustrated later, a much larger and intertwined constellation of hormonal activity is going on that regulates glucose levels than insulin alone. Accordingly, a healthy non-diabetic’s rate of insulin secretion may vary widely, even when exposed to exactly the same conditions (food, exercise, etc) upon successive repetitions. AID systems cannot sense any of these hundreds of other hormones, and glucose levels alone are insufficient for tight control. Just think of cortisol, the one most T1Ds are familiar with. Sensing glucose levels is critical of course, but there is still sufficient error in the holistic ecosystem of the human body that imposes an upper limit on how well an artificial system can perform.
With the limitation that AID algorithms rely solely glucose values, another factor is the error associated with CGM data. Glucose’s kinetic behavior within the body—and within fluids itself—is so volatile, it’s simply not possible to get accurate measurements of SYSTEMIC glucose levels through interstitial tissue. You can get spot readings, but the degree in which these represent systemic glucose levels is highly error-prone. This adds to overall error rate for algorithmic calculation, further imposing upper limit on AID performance.
Insulin has to be administered through interstitial tissue, which introduces a litany of problems, from the mechanical imprecision inherent to insulin pumps, to the physiology of how the body absorbs and distributes insulin once inside. It’s highly erratic, inconsistent, volatile from one moment to the next, and subject to other hormonal activity that may be going on (that there are no sensors to detect and measure). This third factor exacerbates the potential of AID algorithms even further beyond the first two.
Put all three limitations together, researchers in the medical field do not believe that AID systems will be able to perform much better than they do now. Again, many people benefit, but not everyone, and it isn’t likely to get better.
For that, let’s get into the details of the items listed above.
Maintaining glucose levels is physiologically complex
People oversimplify the correlation between blood glucose levels and the need for insulin. Sure, we have rough correlations of glucose levels to units of insulin that we calculate in order to normalize glucose levels. But we T1Ds also know that these rough estimates often don’t work to varying degrees. And this is because more than just insulin is involved in normalizing glucose levels in both diabetics and non-diabetics alike. I cover a lot of this in my article, “Why Controlling Glucose is so Tricky,” where I cite the large constellation of regulatory and counter-regulatory hormones that are expressed when glucose levels move up or down. T1Ds don’t make their own insulin, but we certainly do generate many of those other hormones involved in the regulatory system (even if there’s some dysfunction with some of them under some conditions, like glucagon).
With this in mind, the actual amount of insulin needed to bring glucose levels down is the byproduct of the interactions and behaviors of all those other hormones, and we don’t have the technology to detect (let alone measure) those hormones, let alone understand their kinetics that would allow an algorithm to determine a more precise amount of insulin for any given glucose value (or trend).
The most obvious example of such a hormone that all T1Ds are familiar with—and deplore—is cortisol, the hormone that acts both as an insulin agonist (which inhibits insulin efficacy) and stimulates the production of glucagon in the liver, which stimulates the production of glucose, raising blood sugar levels. This is typically seen as the “dawn effect” in everyone, where glucose levels rise, and along with it, insulin needs. But it also happens with insufficient sleep, daily stresses, medications, certain kinds of foods, and so on.
And that’s just cortisol—T1Ds also suffer from a variety of metabolic dysfunctions that T2Ds have, such as insulin resistance, which itself is a highly complex ecosystem of bad actors. Tissues, lipids, and a host of other factors can affect now just how much insulin the body may need at any given time, but how long that insulin takes to take effect. These values are highly volatile and inconsistent, making a dosing algorithm even more subject to error.
There’s no better way to understand, test and illustrate these situations than by studying healthy non-diabetic subjects and looking at how their own bodies react to changing glucose levels subject to highly controlled conditions.
An example (of many) is this 2015 study from Stanford University, where researchers provided healthy, non-diabetic subjects with CGMs for two weeks, and regularly gave them exactly the same food and activities at the same time each day. Researchers found large variations in blood sugar levels, not just between each other, but the same individuals had erratic patterns. The figure below comes from that study:
Although glucose levels remained “in range,” their patterns were very chaotic, showing how a healthy, non-diabetic individual’s own pancreas struggles to keep glucose levels stable. (Entry criteria for the study required that all subjects be metabolically healthy.)
Most surprising—and critical to the point here—is that the body produces different amounts of insulin at different times to deal with exactly the same food, according to the paper, “Understanding Oral Glucose Tolerance: Comparison of Glucose or Insulin Measurements During the Oral Glucose Tolerance Test with Specific Measurements of Insulin Resistance and Insulin Secretion,” published in Diabetic Medicine.
Both healthy subjects and those with “impaired glucose tolerance” showed variability of the amount of insulin needed to normalize glucose levels by the end of the two hour window, even upon successive tests.
The hypervariability of the human body’s need for particular rates of insulin, irrespective of whether one is a T1D, cannot possibly be predicted by an algorithm whose sole data is glucose alone.
For all of these reasons, the best T1Ds can go by are rough calculations, such as the insulin-to-glucose ratios that we were all taught when we were first diagnosed. It’s imprecise, awful, and highly error-prone, but it’s the best we can get. This is also why experienced T1Ds eventually learn how to refine their dosing on a case-by-case basis in ways that are not “calculable.” We call it “a hunch.” Hence, the expression that self-management is often more an art than a science.
Glucose is highly volatile and difficult to measure, and CGMs have reached their maximum precision
People think that glucose is equally distributed in the body—if they use a blood glucose monitor, whatever that value is, its error rate is still reliable enough to infer dosing decisions. But that assumption about glucose is not true in most real-world conditions. Glucose moves around the body unevenly, congregating in different areas in different concentrations. Furthermore, glucose behavior in fluids is highly dynamic, making it impossible to infer the total systemic glucose levels in the body, which is necessary for refining reliable dosing decisions (even independently of the the hormone behaviors described above).
All this is explained in greater detail in my article, “Continuous Glucose Monitors: Does Better Accuracy Mean Better Glycemic Control?” In short, when glucose levels are above 200 or 250 (mg/dL), CGM “accuracy” rises to 20% and higher per reading. Similarly, when glucose levels move rapidly, CGM error rates exceed 30%.
String together successive readings under rapidly-changing conditions, there’s going to be a lot of erratic values that make it very hard for an algorithm to figure out exactly what’s going on. This graph from that article illustrates the point by comparing output from a Dexcom G6 and a G7, where the G7 is “more accurate.”
Imagine an algorithm trying to figure out the G7 data. It’s just not going to come up with anything sensible in just a few readings. The algorithm has to gather more events in sequence to reduce the randomness and increase confidence in both the total glucose level and the directionality. The problem with that is lost time. If you try to predict a dose too soon, the data is more likely to be wrong. The longer you wait, the more quickly glucose levels get even more out of control, and the opportunity to have done the right dose has long passed.
The G6, by contrast, yields a better estimate of “systemic glucose levels,” allowing for algorithms (and people) to make better dosing decisions, even with its own margin of error. But, this greater precision comes at the cost of potentially failing to detect rapid movements. This leads to the paradox of—and therefore, inconclusiveness—of CGMs as a base technology for ideal glucose measurements. Sure, it’s good, but unless we can detect glucose levels in the main blood supply, there’s an upper limit on how well an algorithm can make use of CGM values.
Insulin infusion through interstitial tissue
This leads to next problem: the administration of insulin itself also has to go through interstitial tissue, which not only creates a delay in time to absorption, but is similarly inconsistent and chaotic as glucose.
This problem only exacerbates the problem with insulin variability cited earlier, where the amount of insulin needed for what might otherwise appear to be identical glucose levels (and rates of rise and fall) from OGTTs (oral glucose tolerance tests) may vary greatly, even among non-diabetic individuals.
In a non-diabetic, the error bars widen dramatically because, unlike non-diabetics, where insulin goes directly into the portal vein, T1D inject insulin through interstitial tissue, which is much slower, and faces all sorts of confounding factors before it gets into the bloodstream. So, when an algorithm determines and administers a dose that it thinks is appropriate for the given glucose level (which itself has high error bars), that insulin isn’t going to take effect for much longer than is needed to maintain control. The algorithm can attempt to compensate for this by dosing higher or lower, but this is where the reliability of glucose patterns comes up again: How can it predict the right dose if the data itself has too much noise?
All this would make it sound like the entire effort is pointless—there’s just too much noise to make it worthwhile.
No, not that—just that the randomness creates errors that put an upper limit on how well they can possibly work. And that’s where we are now. The fact that AID systems are able to achieve A1c levels between 7-8% is pretty remarkable, given the conditions and technologies we have available. We should be glad we have that, as it’s a fantastic benefit to those who cannot or will not manage their own T1D.
The upper bound on AID efficacy
The details to this point illustrate how the human body’s complexity imposes great variability in insulin needs and timing, combined with the high rate of error from CGM data (due to interstitial tissue), combined with the variability of insulin absorption and efficacy when administered through interstitial tissue.
With all that, consider what kinds of insulin estimates an algorithm might recommend. For example, let’s say that it decides that the amount of insulin could be between 4u and 6u for a given set of conditions, such as the current glucose levels, rate and direction of change, time of day, and historical patterns. Given the potential variability factors just described earlier, the estimated dose range between 4u and 6u predict that glucose levels would end up at 100 mg/dL at the end of two hours. Now factor in the error ratio: plus or minus 30 mg/dL. The user might be able to set how tight they want control to be, which imposes risk, but even so, the algorithm really wants to avoid hypoglycemia—especially the severe kind.
Therefore, the algorithm will conservatively choose the lower (safer) end of the insulin calculation: 4u.
Sure, the levels might not drop that low because the algorithm clipped the aggressive dosing. And yes, maybe the luck of the draw means that glucose levels land at 100. Awesome! But it’ll sometimes err in the other direction, where glucose values go to 130. Ok, not bad, certainly, and no harm done.
But spread this scenario out over time, and glucose levels that are much higher—200, 300 and 400. The algorithm might suggest dosing that brings glucose values to the 100-150 range, but the higher you go, the more dangerous higher levels of insulin becomes. So, the conservative “choice” means that, as time goes on, the number of higher glucose levels increases due to the same error ratios.
All this is why A1c levels are highly unlikely to fall much below 7% for those users who are in rather good control on their own, but more likely in the 8% and higher for those whose food consumption and other lifestyle habits keep glucose levels much higher. The algorithm can’t really deal with these elevated glucose levels reliably without risking severe hypoglycemia. And even then, the conservative estimates still can result in these risky scenarios, simply because the body is a very tricky system.
And this is exactly what was found in a study published in the June 2024 issue of Diabetes Care is a paper titled, “Severe Hypoglycemia and Impaired Awareness of Hypoglycemia Persist in People With Type 1 Diabetes Despite Use of Diabetes Technology: Results From a Cross-sectional Survey.” The authors collected data from 2,074 T1Ds, half of whom used AID systems, whereas the rest were non-AID pump systems. Among all, “only 57.7% reported achieving glycemic targets of A1c <7%, but the number of severe hypoglycemic events from automated systems reached 16% for those using AID systems, versus 19% of non-AID pump users.”
Yes, the AID systems performed better than non-AID pumps, but not by a significant margin, and certainly far worse than the authors had expected. Moreover, only 58% were able to achieve 7% — not the outcome the researchers hoped for.
Medical literature reviews
The list of the three hurdles keeping AID systems from improving is drawn from peer-reviewed papers published in medical journals over the years on the challenges facing AID systems. Consensus statements from groups of researchers collaborate to distill their findings.
One example is this article from the December, 2022 issue of Diabetes Care titled, “Automated Insulin Delivery: Benefits, Challenges, and Recommendations. A Consensus Report of the Joint Diabetes Technology Working Group of the European Association for the Study of Diabetes and the American Diabetes Association.” The finely detailed analysis says that, so long as insulin and glucose levels have to use interstitial tissue for both getting glucose levels and administering insulin, the already-chaotic nature of glucose movements cannot be easily predicted.
A year earlier, this 2021 article published in The Lancet titled, “Opportunities and challenges in closed-loop systems in type 1 diabetes,” enumerates largely the same problems.
Go back even further, such as this analysis from 2015 titled, “Combining glucose monitoring and insulin delivery into a single device: current progress and ongoing challenges of the artificial pancreas.” As you can probably guess, the conclusions are largely the same: interstitial tissue and chaotic glucose patterns cannot be overcome with predictive algorithms.
Indeed, google scholar finds similar peer-reviewed reviews dating back to the year 2000 from researchers, scientists, T1D associations, and working groups, and they all come to similar (if not identical) conclusions: Unless we can get around the barrier of interstitial tissues for sensing glucose and delivering insulin, not a whole lot of improvement can ever be realized beyond current AID performance.
Now, again, this doesn’t mean AID systems are not useful, or even good. For many people who can’t achieve those levels on their own, they’re great. So, they should use them, right?
The Moral Hazard
There’s one more issue: The moral hazard.
When using and becoming dependent on automation, one tends to pay less attention to the disease, so people won’t build the experiences that teach those nuances of being self-aware and learning to do better on their own. In fact, a study from the T1D Exchange that examined data over eight consecutive years found that even though pump use increased from 57% in 2010–2012 to 63% in 2016–2018, the authors found that “the average A1c overall had risen from 7.8% to 8.4%,” largely because of disengagement.
The alternative to closed-loop systems is pretty simple: Don’t just leave it all up to the algorithm. Stay engaged. When you eat, tell your pump how many carbs. (Don’t know? Take picture—there are many apps that will tell you the carb count.) About to exercise? Tell it! Is your glucose unexpectedly rising or falling? Try to correct it yourself! AID systems can also operate in semi-automated mode, so there is a path to transition away from automation—is not hard, but it does take self-discipline.
Look, I know it really sucks that the paying close attention to T1D management is time-consuming, nerve-wracking, distracting, overwhelming, and so maddening that you just want to scream. I passed my 50th year with T1D in 2023, and I can easily identify with all those feelings. (See my article, “Why I Haven’t Died Yet: My Fifty Years with Diabetes.”) As I wrote in that article, when I decided to go completely self-reliant on all my dosing decisions—including my basal rates—it took me about a year, which saw my A1c drop from 7.3% to 5.5%, with no severe hypos, TIR of 95%, and no weird diets, carb restrictions, or anything magic. (Not saying it’s for everyone, but it’s more common than people talk about.)
I have nothing against AID systems and pumps. But one just needs to set expectations on their future potential. Until there’s a biological solution (such as beta cell replacement), automated systems should be used by those who really need them: those who cannot or will not take care of themselves, for whatever reason. There’s no shame in that at all. If that’s you, make that decision.
I'm happy to have someone who knows their stuff validate my compulsive checking of CGM and overriding many suggestions of the AID (Dexcom/Omnipod 5). I hoped to avoid calibrating with actual blood tests after the first day of a sensor, but can't. Still more standard deviation in my numbers than I like. The only food whose action I can accurately predict is straight sugar, unfortunately.
I am trying the iLet and it is really hard to have to be less engaged and my numbers are worse, but not terrible. We also need faster insulin in addition to blood glucose numbers.